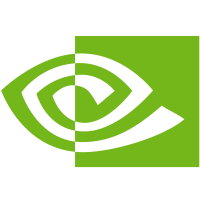
NVIDIA Corp
NASDAQ:NVDA
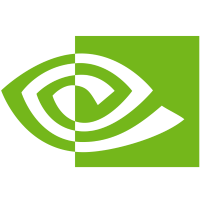
NVIDIA Corp
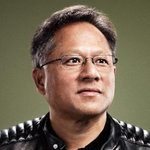
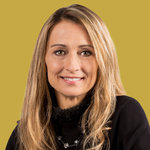
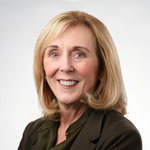
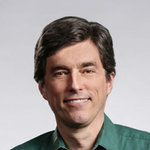
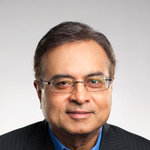
NVIDIA Corporation, founded in 1993 by Jensen Huang, Chris Malachowsky, and Curtis Priem, began its journey with a singular focus on graphics processing units (GPUs) for gaming. This venture coincided with the boom in demand for high-quality visuals, propelling the company to a prominent position in the tech industry. With gaming as its initial stronghold, NVIDIA’s GPUs quickly became the go-to choice for rendering stunning graphics, making the immersive experiences of modern video games possible. However, as technology advanced, so did NVIDIA's appetite for innovation, with the company diversifying its portfolio to apply its GPU technology to artificial intelligence (AI), data centers, and autonomous vehicles. This strategic pivot allowed NVIDIA to transform from a niche player in the gaming industry into a dominant force across multiple high-growth sectors.
Earning revenue primarily through selling its sophisticated GPU hardware and software solutions, NVIDIA capitalizes on several thriving markets. Its gaming segment continues to be a cash cow, fueled by a constant stream of enthusiasts and professionals alike who seek high-performance computing power. Meanwhile, in the data center and AI segments, NVIDIA sells its technology to cloud service providers, enterprises, and researchers eager to harness the power of AI and deep learning. These innovations demand powerful computation capabilities, which NVIDIA’s technology gracefully delivers. The company's reach extends to automation, where it partners with automobile manufacturers to integrate AI systems into vehicles, contributing to the nascent autonomous driving market. Through a blend of hardware prowess and forward-thinking software development, NVIDIA has positioned itself as more than just a graphics chip producer; it’s a versatile tech powerhouse that caters to the future of computing.
Earnings Calls
In Q4, NVIDIA achieved a record revenue of $39.3 billion, up 78% year-on-year, significantly surpassing the expected $37.5 billion. For fiscal 2025, total revenue reached $130.5 billion, a remarkable 114% increase. Data Center revenue alone hit a record $35.6 billion, doubling from the previous year, fueled by strong Blackwell sales which generated $11 billion. The company anticipates Q1 revenue to rise to $43 billion and expects gross margins to stabilize around 71%. NVIDIA's infrastructure is pivotal in accelerating AI capabilities, with projections of a growing incorporation of AI in industries ranging from automotive to healthcare.
Management
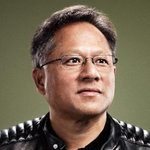
Jen-Hsun (Jensen) Huang is a notable figure in the technology industry, primarily known as the co-founder, president, and CEO of NVIDIA Corporation, a leading company in graphics processing unit (GPU) technologies. Born on February 17, 1963, in Taipei, Taiwan, he moved to the United States at a young age. Huang attended Oregon State University, where he earned a bachelor's degree in electrical engineering. He later received his Master's degree in electrical engineering from Stanford University. Before founding NVIDIA, Huang worked at LSI Logic and Advanced Micro Devices (AMD), gaining significant experience in the semiconductor and computer industries. In 1993, Huang co-founded NVIDIA with Chris Malachowsky and Curtis Priem. Under his leadership, the company has become a dominant force in computer graphics, known for its cutting-edge GPUs that are widely used in gaming, professional visualization, data centers, and increasingly in artificial intelligence (AI) and machine learning applications. NVIDIA's technologies have also been pivotal in the development of self-driving cars and other high-performance computing applications. Huang is admired for his visionary leadership and innovative approach, steering NVIDIA through various technological evolutions. He has been instrumental in positioning NVIDIA at the forefront of AI and GPU advancements, significantly impacting industries beyond gaming, including healthcare, automotive, and manufacturing. His leadership style and strategic acumen have been recognized with numerous awards and honors, including being named one of the world's best CEOs by publications such as Harvard Business Review and Fortune. Huang is also known for his philanthropic efforts, primarily focusing on education and technology initiatives.
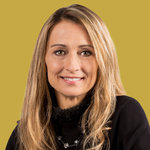
Colette M. Kress is the Executive Vice President and Chief Financial Officer (CFO) of NVIDIA Corporation, a leading technology company known for its graphics processing units (GPUs) and advancements in areas like artificial intelligence and autonomous vehicles. Kress joined NVIDIA in 2013, bringing a wealth of experience in financial management and strategic planning. Before her time at NVIDIA, Kress held significant roles in prominent technology firms. She served as Senior Vice President and CFO of the Business Technology and Operations Finance organization at Cisco Systems, Inc. Her career also includes a tenure at Microsoft Corporation, where she was involved in finance for the Server and Tools division. Additionally, she has worked with Texas Instruments, further expanding her expertise in the tech sector. Kress is widely recognized for her financial acumen and leadership skills, contributing to NVIDIA's growth and success in the technology industry. She plays a crucial role in overseeing the company’s financial strategies, investor relations, and corporate development initiatives. Kress holds a Bachelor’s degree in Arts in Economics from the University of Arizona and an MBA from Southern Methodist University. Her strategic insights and financial stewardship have been instrumental in steering NVIDIA through its continued expansion and innovation.
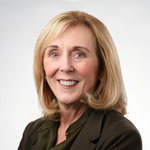
Debora Shoquist serves as the Executive Vice President of Operations at NVIDIA Corporation. In her role, she is responsible for overseeing the company’s global operations, ensuring that NVIDIA's supply chain is robust and efficient to support the company's growth and technological advancements. Under her leadership, the operations team manages procurement, manufacturing, logistics, and quality assurance. Shoquist brings a wealth of experience from her previous roles in the technology industry, contributing significantly to NVIDIA’s infrastructure and operational strategies. Her leadership has been instrumental in supporting the company's innovation trajectory in the competitive tech landscape.
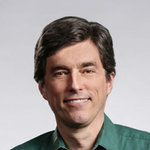
Timothy S. Teter is an accomplished legal professional who serves as the Executive Vice President, General Counsel, and Secretary at NVIDIA Corporation. He joined NVIDIA in 2017, bringing with him extensive experience in corporate law, governance, and intellectual property. Before joining NVIDIA, Teter had a successful legal career at the law firm Cooley LLP, where he was a partner in the litigation department. His practice focused on intellectual property and complex commercial litigation, providing legal strategy and defenses for high-profile technology companies. His work was particularly centered on patent law, making him well-suited for a leading role at NVIDIA, a company deeply involved in cutting-edge technology and innovation. At NVIDIA, Teter leads the company’s global legal matters, overseeing corporate governance, compliance, legal affairs, and intellectual property issues. His strategic leadership in navigating the complex legal landscapes of technology and innovation has been influential in NVIDIA’s growth and success. Teter holds a Juris Doctor degree from Stanford Law School and has a bachelor's degree in mechanical engineering from the University of California, Davis. His technical background in engineering, combined with his legal expertise, enables him to effectively manage and address the multifaceted legal challenges faced by a leading technology company like NVIDIA.
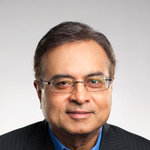
Ajay K. Puri is a long-serving executive at NVIDIA Corporation, where he plays a significant role in the company's operations and business strategies. He has held various leadership positions within the company, contributing to its growth and technological advancements. Ajay Puri is known for his expertise in semiconductor and technology sectors, leveraging his extensive experience to drive NVIDIA’s initiatives in graphics processing units (GPUs) and artificial intelligence (AI). His leadership has been instrumental in expanding NVIDIA's influence across different markets, such as gaming, professional visualization, data centers, and automotive technology. Throughout his tenure, Puri has been recognized for his strategic vision and ability to foster innovation within the company. While specific details about his educational background and early career might not be widely publicized, his impact on NVIDIA's success and reputation as a tech leader is well-regarded in the industry.
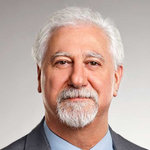
Chris A. Malachowsky is a prominent figure in the technology industry, best known as one of the co-founders of NVIDIA Corporation, which he helped establish in 1993. Malachowsky has played a vital role in shaping NVIDIA into a global leader in graphics processing technology. He co-founded the company alongside Jensen Huang and Curtis Priem, with the ambition of revolutionizing computing graphics and visualization. Malachowsky holds a Bachelor of Science degree in Electrical Engineering from the University of Florida and a Master of Science degree in Computer Science from Santa Clara University. Before founding NVIDIA, he worked at several notable companies such as Hewlett-Packard and Sun Microsystems, where he gained substantial experience and expertise in integrated-circuit design and computer graphics. Within NVIDIA, Malachowsky has held various roles and has been instrumental in driving the company's success through his contributions to engineering and technology development. Known for spearheading advancements in GPU architectures, he has significantly influenced the design and development of some of NVIDIA's most groundbreaking graphics technologies. In addition to his technical contributions, Chris Malachowsky is also recognized for his work in education and philanthropy. He is involved in initiatives that promote STEM education and research, further extending his impact beyond the immediate tech industry.
Donald F. Robertson Jr. is an accomplished legal and corporate governance professional, serving as the Chief Legal Officer and Secretary at NVIDIA Corporation. In his role, Robertson oversees NVIDIA's legal affairs, which encompasses corporate governance, intellectual property, litigation, compliance, and other legal matters critical to the company’s growth and innovation in the technology sector. His leadership and expertise support NVIDIA’s strategic initiatives and ensure adherence to legal and regulatory requirements in the rapidly evolving tech landscape. Robertson's experience and contributions have been integral in navigating complex legal challenges and fostering an environment of compliance and ethical business practices at NVIDIA.
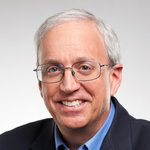
Prof. William J. Dally is a renowned computer scientist and engineer, currently serving as the Chief Scientist and Senior Vice President of Research at NVIDIA Corporation. He is widely recognized for his contributions to computer architecture and parallel computing. Dr. Dally obtained his B.S. in Electrical Engineering from Virginia Tech in 1980, an M.S. from Stanford University in 1981, and a Ph.D. in Computer Science from the California Institute of Technology in 1986. Early in his career, he was involved with the development of high-speed interconnection networks and processing architectures. His work laid the foundation for subsequent advancements in the field of high-performance computing. Before joining NVIDIA, Dr. Dally held academic positions at prestigious institutions such as the Massachusetts Institute of Technology (MIT) and Stanford University. At Stanford, he was the Willard R. and Inez Kerr Bell Professor of Engineering and also served as the Chair of the Computer Science Department. At NVIDIA, Dr. Dally has been instrumental in advancing GPU computing and developing innovative technologies that leverage parallelism to improve computing efficiency and performance. His leadership in research and development has propelled NVIDIA to the forefront of artificial intelligence and graphics technology. In addition to his corporate role, Dr. Dally remains involved in academia, mentoring students and contributing to scholarly research. He has authored numerous publications and holds several patents in the areas of computer architecture and digital systems. Dr. Dally's contributions to the field have been recognized through various awards and honors, reflecting his impact on both theoretical advancements and practical applications in computing.
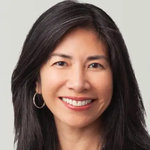
Mylene Mangalindan is an executive at NVIDIA Corporation, a leading technology company known for its graphics processing units (GPUs) and innovative advancements in AI and computing. At NVIDIA, Mangalindan serves as the Head of Internal Communications, where she is responsible for developing and executing communication strategies that engage and inform employees across the organization. Her role involves crafting messages that align with the company's objectives, culture, and vision, ensuring that the internal audience is well-informed about key initiatives and developments. Mangalindan has a rich background in communications, with extensive experience in both corporate and media environments. Before joining NVIDIA, she held various communication roles and was associated with prominent media outlets, where she honed her skills in storytelling and strategic messaging. Her leadership at NVIDIA is characterized by a deep commitment to fostering transparent and effective communication, which is crucial in maintaining a cohesive and motivated workforce, especially in a rapidly evolving tech landscape. Mylene Mangalindan’s role is pivotal in facilitating the flow of information within NVIDIA, contributing to the company's continued innovation and success.

Tommy Lee is a notable executive at NVIDIA Corporation, a leading company in the field of graphics processing units (GPUs) and AI technology. He currently serves in the capacity of Vice President, Embedded and Edge Computing. In his role, he is responsible for overseeing the strategic development and deployment of NVIDIA’s embedded systems and edge computing solutions, which are pivotal for applications in various industries including automotive, robotics, and IoT devices. Lee holds a significant background in technology and business management, having accumulated years of experience in driving innovation and leading high-performing teams. His leadership at NVIDIA is instrumental in expanding the company's computing platforms beyond PCs and data centers, bringing sophisticated AI capabilities to edge devices, which operate in application environments where low latency and high efficiency are critical. Throughout his career, Tommy Lee has been recognized for his contributions to advancing the integration of AI in everyday technology.
Good afternoon. My name is Krista, and I will be your conference operator today. At this time, I would like to welcome everyone to NVIDIA's fourth quarter earnings call. [Operator Instructions]. Thank you, Stewart Stecker, you may begin your conference.
Thank you. Good afternoon, everyone, and welcome to NVIDIA's conference call for the fourth quarter of fiscal 2025. With me today from NVIDIA are Jensen Huang, President and Chief Executive Officer; and Colette Kress, Executive Vice President and Chief Financial Officer.
I'd like to remind you that our call is being webcast live on NVIDIA's Investor Relations website. The webcast will be available for replay until the conference call to discuss our financial results for the first quarter of fiscal 2026. The content of today's call is NVIDIA's property. It can't be reproduced or transcribed without prior written consent.
During this call, we may make forward-looking statements based on current expectations. These are subject to a number of significant risks and uncertainties, and our actual results may differ materially. For a discussion of factors that could affect our future financial results and business, please refer to the disclosure in today's earnings release, our most recent forms 10-K and 10-Q and the reports that we may file on Form 8-K with the Securities and Exchange Commission.
All our statements are made as of today, February 26, 2025, based on information currently available to us. Except as required by law, we assume no obligation to update any such statements.
During this call, we will discuss non-GAAP financial measures. Confine a reconciliation of these non-GAAP financial measures to GAAP financial measures in our CFO commentary, which is posted on our website.
With that, let me turn the call over to Colette.
Thanks, Stewart. Q4 was another record quarter. Revenue of $39.3 billion was up 12% sequentially and -- and up 78% year-on-year and above our outlook of $37.5 billion. For fiscal 2025 revenue was $130.5 billion, up 114% from the prior year.
Let's start with Data Center. Data center revenue for fiscal 2025 was $115.2 billion, more than doubling from the prior year. In the fourth quarter, Data Center revenue of $35.6 billion was a record, up 16% sequentially and 93% year-on-year. As the Blackwell ramp commenced and Hopper 200 continued sequential growth.
In Q4, Blackwell sales exceeded our expectations. We delivered $11 billion of Blackwell revenue to meet strong demand. This is the fastest product ramp in our company's history, unprecedented in its speed and scale. Blackwell production is in full year across multiple configurations, and we are increasing supply quickly expanding customer adoption.
Our Q4 Data Center compute revenue jumped 18% sequentially and over 2x year-on-year. Customers are racing to scale infrastructure to train the next generation of cutting-edge models and unlock the next level of AI capabilities. With Blackwell, it will be common for these clusters to start with 100,000 GPUs or more. Shipments have already started for multiple infrastructures of this size.
Post training and model customization are fueling demand for NVIDIA infrastructure and software as developers and enterprises leverage techniques such as fine-tuning reinforcement learning and distillation to tailor models for domain-specific use cases. Hugging Face alone hosts over 90,000 derivatives freighted from the Llama foundation model.
The scale of post-training and model customization is massive and can collectively demand orders of magnitude, more compute than pretraining.
Our inference demand is accelerating, driven by test time scaling and new reasoning models like OpenAI, [ Postgre], DeepSeek-R1 and Grok 3. Long thinking reasoning AI can require 100x more compute per task compared to 1 shot inferences. Blackwell was architected for reasoning AI inference. Blackwell supercharges reasoning AI models with up to 25x higher token throughput and 20x lower cost versus Hopper 100. It is revolutionary transformer engine is built for LLM and mixture of experts inference.
And its NVLink Domain delivers 14x the throughput of PCIe Gen 5, ensuring the response time, throughput and cost efficiency needed to tackle the growing complexity of infants of scale.
Companies across industries are tapping into NVIDIA's whole STAG inference platform to boost performance and slash costs. Now tripled inference throughput and cut costs by 66%, using NVIDIA TensorRT for its screenshot feature. Perplexity sees 435 million monthly queries and reduced its inference costs, 3 with NVIDIA Triton Infant Server and TensorRT LLM.
Microsoft Bing achieved a 5x speed up at major TCO savings for visual search across billions of images with NVIDIA, TensorRT and acceleration libraries.
Blackwell has great demand for inference. Many of the early GB200 deployments are earmarked for inference, a first for a new architecture. Blackwell addresses the entire AI market from pretraining, post training to inference across cloud, to on-premise, to enterprise. CUDA's programmable architecture accelerates every AI model and over 4,400 applications, ensuring large infrastructure investments against obsolete in rapidly evoluting market.
Our performance and pace of innovation is unmatched. We're driven to a 200x reduction in inference costs in just the last 2 years. We delivered the lowest TCO and the highest ROI. And full stack optimizations for NVIDIA and our large ecosystem, including 5.9 million developers continuously improve our customers' economics.
In Q4, large CSPs represented about half of our data center revenue. and these sales increased nearly 2x year-on-year. Large CSPs were some of the first to stand up Blackwell with Azure, GCP, AWS and OCI bringing GB200 systems to cloud regions around the world to meet certain surging customer demand for AI.
Regional cloud hosting NVIDIA GPUs increased as a percentage of data center revenue, reflecting continued AI factory build-outs globally and rapidly rising demand for AI reasoning models and agents. We've launched a 100,000 GB200 cluster-based incidents with NVLink Switch and Quantum 2 InfiniBand.
Consumer Internet revenue grew 3x year-on-year, driven by an expanding set of generative AI and deep learning use cases. These include recommender systems, vision, language understanding, synthetic data generation search and Agentic AI. For example, xAI is adopting the GB200 to train and inference its next generation of Grok AI models. Meta's cutting edge Andromeda advertising engine runs on NVIDIA's Grace Hopper Superchip serving vast quantities of ads across Instagram, Facebook applications.
Andromeda harnesses Grace Hopper's fast interconnect and large memory to boost inference throughput by 3x, enhanced ad personalization and deliver meaningful jumps in monetization and ROI.
Enterprise revenue increased nearly 2x year on accelerating demand for model fine-tuning, RAG and Agentic AI workflows and GPU accelerated data processing. We introduced NVIDIA Llama Nemotron model family NIMs to help developers create and deploy AI agents across a range of applications including customer support, fraud detection and product supply chain and inventory management.
Leading AI agent platform providers, including SAP and ServiceNow are among the first to use new models. Health care leaders IQVIA, Illumina, Mayo Clinic and Arc Institute are using NVIDIA AI to speed drug discovery enhanced genomic research and Pioneer advanced health care services with generative and Agentic AI.
As AI expands beyond the digital world, NVIDIA infrastructure and software platforms are increasingly being adopted to power robotics and physical AI development. One of the early and largest robotics applications and autonomous vehicles where virtually every AV company is developing on NVIDIA in the data center, the car or both.
NVIDIA's automotive vertical revenue is expected to grow to approximately $5 billion this fiscal year. At CES, Hyundai Motor Group announced it is adopting NVIDIA technologies to accelerate AV and robotics development and smart factory initiatives.
Vision transformers, self supervised learning, multimodal sensor fusion and high fidelity simulation are driving breakthroughs in AV development and will require 10x more compute. At CES, we announced the NVIDIA COSMO World Foundation model platform. Just as language, foundation models have revolutionized Language AI, Cosmos is a physical AI to revolutionize robotics. The robotics and automotive companies, including ridesharing giant Uber, are among the first to adopt the platform.
From a geographic perspective, sequential growth in our Data Center revenue was strongest in the U.S., driven by the initial ramp up Blackwell. Countries across the globe are building their AI ecosystem as demand for compute infrastructure is surging. France's EUR 100 billion AI investment and the EU's EUR 200 billion invest AI initiatives offer a glimpse into the build-out to set redefined global AI infrastructure in the coming years.
Now as a percentage of total Data Center revenue, data center sales in China remained well below levels seen on the onset of export controls. Absent any change in regulations, we believe that China shipments will remain roughly at the current percentage. The market in China for data center solutions remains very competitive. We will continue to comply with export controls while serving our customers.
Networking revenue declined 3% sequentially. Our networking attached to GPU compute systems is robust at over 75%. We are transitioning from small NVLink 8 with InfiniBand, to large NVLink 72 with Spectrum-X. Spectrum-X and NVLink Switch revenue increased and represents a major new growth vector. We expect networking to return to growth in Q1.
AI requires a new class of networking. NVIDIA offers NVLink Switch systems for scale-up compute. For scale out, we offer quantum incentive for HPC supercomputers and Spectrum X for Ethernet environments. Spectrum-X enhances the Ethernet for AI computing and has been a huge success. Microsoft Azure, OCI, CoreWeave and others are building large AI factories with Spectrum-X.
The first Stargate data centers will use Spectrum-X. Yesterday, Cisco announced integrating Spectrum-X into their networking portfolio to help enterprises build AI infrastructure. With its large enterprise footprint and global reach, Cisco will bring NVIDIA Ethernet to every industry.
Now moving to gaming and ARPCs. Gaming revenue of $2.5 billion decreased 22% sequentially and 11% year-on-year. Full year revenue of $11.4 billion increased 9% year-on-year, and demand remains strong throughout the holiday. However, Q4 shipments were impacted by supply constraints. We expect strong sequential growth in Q1 as supply increases.
The new GeForce RTX 50 Series desktop and laptop GPUs are here. Build for gamers, creators and developers they fuse AI and graphics redefining visual computing, powered by the Blackwell architecture, fifth generation Tensor cores and fourth-generation RT cores and featuring UQ's400AI tops. These GPUs deliver a 2x performance leap and new AI-driven rendering including neuro shaders, digital human technologies, geometry and lighting.
The new DLSS 4 boost frame rates up to 8x with AI-driven frame generation, turning 1 rendered frame into 3. It also features the industry's first real-time application of transformer models packing 2x more parameters and 4x to compute for unprecedented visual fidelity.
We also announced a wave of GeForce Blackwell laptop GPUs with new NVIDIA Max-Q technology that extends battery life by up to an incredible 40%. And -- these laptops will be available starting in March from the world's top manufacturers.
Moving to our professional visualization business. Revenue of $511 million was up 5% sequentially and 10% year-on-year. Full year revenue of $1.9 billion increased 21% year-on-year. Key industry verticals driving demand include automotive and health care.
NVIDIA Technologies and generative AI are reshaping design, engineering and simulation workloads. Increasingly, these technologies are being leveraged in leading software platforms from ANSYS, Cadence and Siemens fueling demand for NVIDIA RTX workstations.
Now moving to Automotive. Revenue was a record $570 million, up 27% sequentially and up 103% year-on-year. Full year revenue of $1.7 billion increased 5% year-on-year. Strong growth was driven by the continued ramp in autonomous vehicles, including cars and robotaxis. At CES, we announced Toyota, the world's largest auto maker will build its next-generation vehicles on NVIDIA Orin running the safety certified NVIDIA DriveOS. We announced Aurora and Continental will deploy driverless trucks at scale powered by NVIDIA Drive Thor.
Finally, our end-to-end autonomous vehicle platform NVIDIA Drive Hyperion has passed industry safety assessments like TĂśV SUD and TUV Rheinland, 2 of the industry's foremost authorities for automotive grade safety and cybersecurity. NVIDIA is the first AV platform that received a comprehensive set of third-party assessments.
Okay. Moving to the rest of the P&L. GAAP gross margin was 73% and non-GAAP gross margin was 73.5% and down sequentially as expected with our first deliveries of the Blackwell architecture. As discussed last quarter, Blackwell is a customizable AI infrastructure with several different types of NVIDIA build chips multiple networking options and for air and liquid-cooled data center.
We exceeded our expectations in Q4 in ramping Blackwell, increasing system availability, providing several configurations to our customers. As Blackwell ramps, we expect gross margins to be in the low 70s.
We -- initially, we are focused on expediting the manufacturing of Blackwell systems to meet strong customer demand as they race to build out Blackwell infrastructure. When fully ramped, we have many opportunities to improve the cost and gross margin will improve and return to the mid-70s, late this fiscal year.
Sequentially, GAAP operating expenses were up 9% and non-GAAP operating expenses were 11%, reflecting higher engineering development costs and higher compute and infrastructure costs for new product introductions. In Q4, we returned $8.1 billion to shareholders in the form of share repurchases and cash dividends.
Let me turn to the outlook in the first quarter. Total revenue is expected to be $43 billion, plus or minus 2%. And -- Continuing with its strong demand, we expect a significant ramp of Blackwell in Q1. We expect sequential growth in both Data Center and Gaming. Within Data Center, we expect sequential growth from both compute and networking.
GAAP and non-GAAP gross margins are expected to be 70.6% and 71%, respectively, plus or minus 50 basis points. GAAP and non-GAAP operating expenses are expected to be approximately $5.2 billion and $3.6 billion, respectively. We expect full year fiscal year '26 operating expenses to grow to be in the mid-30s.
GAAP and non-GAAP other incoming expenses are expected to be an income of approximately $400 million. excluding gains and losses from nonmarketable and publicly held equity securities. GAAP and non-GAAP tax rates are expected to be 17%, plus or minus 1%, excluding any discrete items.
Further financial details are included in the CFO commentary and other information available on our IR website, including a new financial information AI agent.
In closing, let me highlight upcoming events for the financial community. We will be at the TD Cowen Health Care Conference in Boston on March 3 and at the Morgan Stanley Technology, Media and Telecom Conference in San Francisco on March 5. Please join us for our Annual GTC conference starting Monday, March 17 in San Jose, California. Jensen will deliver a news-packed keynote on March 18, and we will host a Q&A session for our financial analysts for the next day, March 19. We look forward to seeing you at these events. Our earnings call to discuss the results for our first quarter of fiscal 2026 is scheduled for May 28, 2025.
I -- we are going to open up the call, operator, to questions. If you could start that, that would be great.
[Operator Instructions]. And your first question comes from CJ Muse with Cantor Fitzgerald.
I guess for me, Jensen, as [indiscernible] compute and reinforcement learning shows such promise, we're clearly seeing an increasing blurring of the lines between training and inference, -- what does this mean for the potential future of potentially inference dedicated clusters? And how do you think about the overall impact to NVIDIA and your customers?
Yes, I appreciate that C.J. There are now multiple scaling loss. There's the pre-training scaling law, and that's going to continue to scale because we have multimodality, we have data that came from reasoning that are now used to do pretraining.
And then the second is post-training skilling, using reinforcement learning human feedback, reinforcement learning AI feedback, reinforcement learning, verifiable rewards. The amount of computation you use for post training is actually higher than pretraining. And it's kind of sensible in the sense that you could, while you're using reinforcement learning, generate an enormous amount of synthetic data or synthetically generated tokens. AI models are basically generating tokens to train AI models. And that's post-trade.
And the third part, this is the part that you mentioned is test time compute or reasoning, long thinking, new [indiscernible] scaling. They're all basically the same ideas. And there you have a chain of thought, you've search. The amount of tokens generated the amount of inference compute needed is already 100x more than the one-shot examples and the one-shot capabilities of large language models in the beginning. And that's just the beginning. This is just the beginning.
The idea that the next generation could have thousands times and even hopefully, extremely thoughtful and simulation-based and search-based models that could be hundreds of thousands, millions of times more compute than today is in our future.
And so the question is how do you design such an architecture? Some of it -- some of the models are auto regressive. Some of the models are diffusion based. Some of it -- some of the times you want your data center to have disaggregated inference. Sometimes it is compacted.
And so it's hard to figure out what is the best configuration of a data center, which is the reason why NVIDIA's architecture is so popular. We run every model. We are great at training. The vast majority of our compute today is actually inference and Blackwell takes all of that to a new level. We designed Blackwell with the idea of reasoning models in mind. And when you look at training, it's many times more performing.
But what's really amazing is for long thinking test time scaling, reasoning AI models were tens of times faster, 25x higher throughput. And so Blackwell is going to be incredible across the board. And when you have a data center that allows you to configure and use your data center based on are you doing more pretraining now, post training now or scaling out your inference, our architecture is fungible and easy to use in all of those different ways. And so we're seeing, in fact, much, much more concentration of a unified architecture than ever before.
Your next question comes from the line of Joe Moore with JPMorgan.
Morgan Stanley, actually. I wonder if you could talk about GB200 at CES, you sort of talked about the complexity of the rack level systems and the challenges you have. And then as you said in the prepared remarks, we've seen a lot of general availability -- where are you in terms of that ramp?
Are there still bottlenecks to consider at a systems level above and beyond the chip level? And just have you maintained your enthusiasm for the NVL72 platforms?
Well, I'm more enthusiastic today than I was at CES. And the reason for that is because we've shipped a lot more since CES. We have some 350 plants manufacturing the 1.5 million components that go into each one of the Blackwell racks, Grace Blackwell racks.
Yes, it's extremely complicated. And we successfully and incredibly ramped up Grace Blackwell, delivering some $11 billion of revenues last quarter. We're going to have to continue to scale as demand is quite high, and customers are anxious and impatient to get their Blackwell systems.
You've probably seen on the web, a fair number of celebrations about Grace Blackwell systems coming online and we have them, of course. We have a fairly large installation of Grace Blackwell goes for our own engineering and our own design teams and software teams.
CoreWeave has now been quite public about the successful bring up of theirs. Microsoft has, of course, open AI has, and you're starting to see many come online. And so I think the answer to your question is nothing is easy about what we're doing, but we're doing great, and all of our partners are doing great.
Your next question comes from the line of Vivek Arya with Bank of America Securities.
Colette if you wouldn't mind confirming if Q1 is the bottom for gross margins? And then Jensen, my question is for you. What is on your dashboard to give you the confidence that the strong demand can sustain into next year? And has DeepSeek and whatever innovations they came up with, has that changed that view in any way? .
Let me first take the first part of the question there regarding the gross margin. During our Blackwell ramp, our gross margins will be in the low 70s. At this point, we are focusing on expediting our manufacturing, expediting our manufacturing to make sure that we can provide to customers as soon as possible. Our Blackwell is fully round. And once it does -- I'm sorry, once our Blackwell fully rounds, we can improve our cost and our gross margin. So we expect to probably be in the mid-70s later this year.
Walking through what you heard Jensen speak about the systems and their complexity, they are customizable in some cases. They've got multiple networking options. They have liquid cool and water cooled. So we know there is an opportunity for us to improve these gross margins going forward. But right now, we are going to focus on getting the manufacturing complete and to our customers as soon as possible.
We know several things that we have a fairly good line of sight of the amount of capital investment that data centers are building out towards. We know that going forward, the vast majority of software is going to be based on machine learning. And so accelerated computing and generative AI, reasoning AI are going to be the type of architecture you want in your data center. .
We have, of course, forecast and plans from our top partners. And we also know that there are many innovative, really exciting start-ups that are still coming online as new opportunities for developing the next breakthroughs in AI, whether it's Agentic AIs, reasoning AI or physical AIs. The number of start-ups are still quite vibrant and each 1 of them need a fair amount of computing infrastructure.
And so I think the -- whether it's the near term signals or the midterm signals, near-term signals, of course, are POs and forecasts and things like that. Midterm signals would be the level of infrastructure and CapEx scale-out compared to previous years. And then the long-term signals has to do with the fact that we know fundamentally software has changed from hand coding that runs on CPUs, to machine learning and AI-based software that runs on GPUs and accelerated computing systems.
And so we have a fairly good sense that this is the future of software. And then maybe as you roll it out, another way to think about that is we've really only tapped consumer AI and search and some amount of consumer generative AI, advertising, recommenders, kind of the early days of software. The next wave is coming, Agentic AI for enterprise, physical AI for robotics and sovereign AI as different regions build out their AI for their own ecosystems.
And so each one of these are barely off the ground, and we can see them. We can see them because, obviously, we're in the center of much of this development and we can see great activity happening in all these different places and these will happen. So near term, midterm, long term.
Your next question comes from the line of Harlan Sur with JPMorgan.
Your next-generation Blackwell Ultra is set to launch in the second half of this year, in line with the team's annual product cadence. Jensen, can you help us understand the demand dynamics for Ultra given that you'll still be ramping the current generation Blackwell solutions? How do your customers and the supply chain also manage the simultaneous ramps of these 2 products? And -- is the team still on track to execute Blackwell Ultra in the second half of this year? .
Yes. Blackwell Ultra is second half. As you know, the first Blackwell was we had a hiccup that probably cost us a couple of months. We're fully recovered, of course. The team did an amazing job recovering and all of our supply chain partners and just so many people helped us recover at the speed of light. And so now we've successfully ramped production of Blackwell.
But that doesn't stop the next train. The next train is on an annual rhythm and Blackwell Ultra with new networking, new memories and of course, new processors, and all of that is coming online. We've have been working with all of our partners and customers, laying this out. They have all of the necessary information, and we'll work with everybody to do the proper transition.
This time between Blackwell and Blackwell Ultra, the system architecture is exactly the same. It's a lot harder going from Hopper to Blackwell because we went from an NVLink 8 system to a NVLink 72-based system. So the chassis, the architecture of the system, the hardware, the power delivery, all of that had to change. This was quite a challenging transition.
But the next transition will slot right in Blackwell Ultra will slot right in. We've also already revealed and been working very closely with all of our partners on the click after that. And the click after that is called Vera Rubin and all of our partners are getting up to speed on the transition of that and so preparing for that transition. And again, we're going to provide a big, huge step-up. And so come to GTC, and I'll talk to you about Blackwell Ultra, Vera Rubin and then show you what we place after that. Really exciting new products, so to come to GTC piece.
Your next question comes from the line of Timothy Arcuri with UBS.
Jensen, we heard a lot about custom ASICs. Can you kind of speak to the balance between customer ASIC and merchant GPU. We hear about some of these heterogeneous superclusters to use both GPU and ASIC? Is that something customers are planning on building? Or will these infrastructures remain fairly distinct .
Well, we built very different things than ASICs, in some ways, completely different in some areas we intercept. We're different in several ways. One, NVIDIA'S architecture is general whether you're -- you've optimized for unaggressive models or diffusion-based models or vision-based models or multimodal models or text models. We're great in all of it. .
We're great on all of it because our software stack is so -- our architecture is sensible, our software stack ecosystem is so rich that were the initial target of most exciting innovations and algorithms. And so by definition, we're much, much more general than narrow. We're also really good from the end-to-end from data processing, the curation of the training data, to the training of the data, of course, to reinforcement learning used in post training, all the way to inference with tough time scaling.
So we're general, we're end-to-end, and we're everywhere. And because we're not in just one cloud, we're in every cloud, we could be on-prem. We could be in a robot. Our architecture is much more accessible and a great target initial target for anybody who's starting up a new company. And so we're everywhere.
And the third thing I would say is that our performance in our rhythm is so incredibly fast. Remember that these data centers are always fixed in size. They're fixed in size or they're fixing power. And if our performance per watt is anywhere from 2x to 4x to 8x, which is not unusual, it translates directly to revenues. And so if you have a 100-megawatt data center, if the performance or the throughput in that 100-megawatt or the gigawatt data center is 4x or 8x higher, your revenues for that gigawatt data center is 8x higher.
And the reason that is so different than data centers of the past is because AI factories are directly monetizable through its tokens generated. And so the token throughput of our architecture being so incredibly fast is just incredibly valuable to all of the companies that are building these things for revenue generation reasons and capturing the fast ROI. And so I think the third reason is performance.
And then the last thing that I would say is the software stack is incredibly hard. Building an ASIC is no different than what we do. We build a new architecture. And the ecosystem that sits on top of our architecture is 10x more complex today than it was 2 years ago. And that's fairly obvious because the amount of software that the world is building on top of architecture is growing exponentially and AI is advancing very quickly.
So bringing that whole ecosystem on top of multiple chips is hard. And so I would say that those 4 reasons. And then finally, I will say this, just because the chip is designed doesn't mean it gets deployed. And you've seen this over and over again. There are a lot of chips that gets built, but when the time comes, a business decision has to be made, and that business decision is about deploying a new engine, a new processor into a limited AI factory in size, in power and in fine.
And our technology is not only more advanced, more performance, it has much, much better software capability and very importantly, our ability to deploy is lightning fast. And so these things are enough for the faint of heart, as everybody knows now. And so there's a lot of different reasons why we do well, why we win.
Your next question comes from the line of Ben Reitzes with Melius Research.
Jensen, it's a geography-related question. you did a great job explaining some of the demand underlying factors here on the strength. But U.S. was up about $5 billion or so sequentially. And I think there is a concern about whether U.S. can pick up the slack if there's regulations towards other geographies. And I was just wondering, as we go throughout the year, if this kind of surge in the U.S. continues and it's going to be -- whether that's okay. And if that underlies your growth rate, how can you keep growing so fast with this mix shift towards the U.S.? Your guidance looks like China is probably up sequentially. So just wondering if you could go through that dynamic and maybe collect can weigh in. .
China is approximately the same percentage as Q4 and as previous quarters. It's about half of what it was before the export control. But it's approximately the same in percentage.
With respect to geographies, the takeaway is that AI is software. It's modern software. It's incredible modern software, but it's modern software and AI has gone mainstream. AI is used in delivery services everywhere, shopping services everywhere. If you were to buy a quarter from milk is delivered to you, AI was involved.
And so almost everything that a consumer service provides AIs at the core of it. Every student will use AI as a tutor, health care services use AI, financial services use AI. No fintech company will not use AI. Every Fintech company will. Climate tech company use AI. Mineral discovery now uses AI. The number of -- every higher education, every university uses AI and so I think it is fairly safe to say that AI has gone mainstream and that it's being integrated into every application.
And -- and our hope is that, of course, the technology continues to advance safely and advance in a helpful way to society. And with that, we're -- I do believe that we're at the beginning of this new transition.
And what I mean by that in the beginning is, remember, behind us has been decades of data centers and decades of computers that have been built. And they've been built for a world of hand coding and general purpose computing and CPUs and so on and so forth. And going forward, I think it's fairly safe to say that world is going to be almost all software to be infused with AI. All software and all services will be based on -- ultimately, based on machine learning, the data flywheel is going to be part of improving software and services and that the future computers will be accelerated, the future computers will be based on AI.
And we're really 2 years into that journey. And in modernizing computers that have taken decades to build out. And so I'm fairly sure that we're in the beginning of this new era.
And then lastly, no technology has ever had the opportunity to address a larger part of the world's GDP than AI. No software tool ever has.
And so this is now a software tool that can address a much larger part of the world's GDP more than any time in history. And so the way we think about growth and the way we think about whether something is big or small, has to be in the context of that. And when you take a step back and look at it from that perspective, we're really just in the beginning. .
Your next question comes from the line of Aaron Rakers with Wells Fargo.
Your next question comes from Mark Lipacis with Evercore ISI.
I had a clarification and a question. Colette up for the clarification. Did you say that enterprise within the data center grew 2x year-on-year for the January quarter? And if so, does that -- would that make it the fast faster growing than the hyperscalers?
And then, Jensen, for you, the question, hyperscalers are the biggest purchasers of your solutions, but they buy equipment for both internal and external workloads, external workflows being cloud services that enterprise is used. So the question is, can you give us a sense of how that hyperscaler spend splits between that external workload and internal? And -- and as these new AI workflows and applications come up, would you expect enterprises to become a larger part of that consumption mix? And does that impact how you develop your service, your ecosystem.
Sure. Thanks for the question regarding our Enterprise business. Yes, it grew to and very similar to what we were seeing with our large CSPs. Keep in mind, these are both important areas to understand working with the CSPs and be working on large language models, can you working on inference in their own work. But keep in mind, that is also where the enterprises are servicing. Your enterprises are both with your CSPs as well as in terms of building on their own. They're both are growing quite well.
The CSPs are about half of our business. And the CSPs have internal consumption and external consumption, as you say. And we're using -- of course, used for internal consumption. We work very closely with all of them to optimize workloads that are internal to them, because they have a large infrastructure of NVIDIA gear that they could take advantage of.
And the fact that we could be used for AI on the one hand, video processing on the other hand, data processing like Spark, we're fungible. And so the useful life of our infrastructure is much better. If the useful life is much longer, then the TCO is also lower.
And so -- the second part is how do we see the growth of enterprise or not CSPs, if you will, going forward? And the answer is, I believe, long term, it is by far larger and the reason for that is because if you look at the computer industry today and what is not served by the computer industry is largely industrial.
So let me give you an example. When we say enterprise, and let's use the car company as an example because they make both soft things and hard things. And so in the case of a car company, the employees will be what we call enterprise and agenetic AI and software planning systems and tools, and we have some really exciting things to share with you guys at GTC, build Agentic systems are for employees to make employees more productive to design to market plan to operate their company. That's Agenetic AI.
On the other hand, the cars that they manufacture also need AI. They need an AI system that trains the cars, treats this entire giant fleet of cars. And today, there's 1 billion cars on the road. Someday, there will be 1 billion cars on the road, and every single one of those cars will be robotic cars, and they'll all be collecting data, and we'll be improving them using an AI factory. Whereas they have a our factory today in the future they'll have a car factory and an AI factory.
And then inside the car itself is a robotic system. And so as you can see, there are 3 computers involved and there's the computer that helps the people. There's the computer that build the AI for the machineries that could be, of course, could be a tractor, it could be a lawn mower. It could be a human or robot that's being developed today. It could be a building, it could be a warehouse.
These physical systems require new type of AI we call physical AI. They can't just understand the meaning of words and languages, but they have to understand the meaning of the world, friction and inertia, object permanence and cause and effect. And all of those type of things that are common sense to you and I, but AIs have to go learn those physical effects. So we call that physical AI.
That whole part of using Agentic AI to revolutionize the way we work inside companies, that's just starting. This is now the beginning of the agent AI era, and you hear a lot of people talking about it and we got some really great things going on. And then there's the physical AI after that, and then there are robotic systems after that.
And so these 3 computers are all brand new. And my sense is that long term, this will be by far the larger of a mall, which kind of makes sense. The world's GDP is representing -- represented by either heavy industries or industrials and companies that are providing for those.
Your next question comes from the line of Aaron Rakers with Wells Fargo.
Jensen, I'm curious as we now approach the 2-year anniversary of really the Hopper inflection that you saw in 2023 in Gen AI in general. And when we think about the road map you have in front of us, how do you think about the infrastructure that's been deployed from a replacement cycle perspective? And whether if it's GB300 or if it's the Rubin cycle where we start to see maybe some refresh opportunity. I'm just curious how you look at that. .
I appreciate it. First of all, people are still using Voltus and Pascal and amperes. And the reason for that is because there are always things that because CUDA is so programmable you could use it Blackwell, one of the major use cases right now is data processing and data curation. You find a circumstance that an AI model is not very good at. You present that circumstance to a vision language model, let's say, it's a car. You present that circumstance to a vision language model.
The vision language model actually looks in the circumstances, said, this is what happened and I was very good at it. You then take that response to the prompt and you go and prompt an AI model to go find in your whole lake of data other circumstances like that, whatever that circumstance was. And then you use an AI to do domain randomization and generate a whole bunch of other examples.
And then from that, you can go train the bottle. And so you could use an peers to go and do data processing and data curation and machine learning-based search. And then you create the training data set, which you then present to your Hopper systems for training.
And so each one of these architectures are completely -- they're all CUDA-compatible and so everything wants on everything. But if you have infrastructure in place, then you can put the less intensive workloads onto the installed base of the past. All of [indiscernible] very well employed.
We have time for one more question, and that question comes from Atif Malik with Citi.
I have a follow-up question on gross margins for Colette. So I understand there are many moving parts the Blackwell yields, NVLink 72 and Ethernet mix. And you kind of tipped to the earlier question, the April quarter is the bottom,; but second half would have to ramp like 200 basis points per quarter to get to the mid-70s range that you're giving for the end of the fiscal year. And we still don't know much about tariff impact to broader semiconductor. So what kind of gives you the confidence in that trajectory in the back half of this year?
Yes. Thanks for the question. Our gross margins, they're quite complex in terms of the material and everything that we put together in a Blackwell system, a tremendous amount of opportunity to look at a lot of different pieces of that on how we can better improve our gross margins over time. .
Remember, we have many different configurations as well on Blackwell that will be able to help us do that. So together, working after we get some of these really strong ramping completed for our customers, we can begin a lot of that work. If not, we're going to probably start as soon as possible if we can. If we can improve it in the short term, we will also do that.
Tariff at this point, it's a little bit of an unknown it's an unknown until we understand further what the U.S. government's plan is, both its timing, it's where and how much. So at this time, we are awaiting, but again, we would, of course, always follow export controls and/or tariffs in that manner.
Ladies and gentlemen, that does conclude our question-and-answer session. I'm sorry.
Thank you.
We are going to open up to Jensen [indiscernible] a couple of things.
I just wanted to thank you. Thank you, Colette. The demand for Blackwell is extraordinary. AI is evolving beyond perception and generative AI into reasoning. With resenting AI, we're observing another scaling law, inference time or test time scaling, more computation.
The more the model thinks the smarter the answer. Models like OpenAI, Grok 3, DeepSeek-R1 are reasoning models that apply inference time scaling. Reasoning models can consume 100x more compute. Future reasoning models can consume much more compute. DeepSeek-R1 has ignited global enthusiast -- it's an excellent innovation. But even more importantly, it has open source a world-class reasoning AI model.
Nearly every AI developer is applying R1 or chain of thought and reinforcement learning techniques like R1 to scale their model's performance.
We now have 3 scaling laws, as I mentioned earlier, driving the demand for AI computing. The traditional scaling loss of AI remains intact. Foundation models are being enhanced with multimodality, and pretraining is still growing. But it's no longer enough. We have 2 additional scaling dimensions.
Post-training skilling, where reinforcement learning, fine-tuning, model distillation require orders of magnitude more compute than pretraining alone. Inference time scaling and reasoning where a single query and demand 100x more compute. We defined Blackwell for this moment, a single platform that can easily transition from pre-trading, post training and test time scaling.
Blackwell's FP4 transformer engine and NVLink 72 scale-up fabric and new software technologies led Blackwell process reasoning AI models, 25x faster than Hopper. Blackwell in all of this configuration is in full production. Each Grace Blackwell NVLink 72 rack is an engineering marvel. 1.5 million components produced across 350 manufacturing sites by nearly 100,000 factory operators.
AI is advancing at life speed. We're at the beginning of reasoning AI and inference time scaling. But we're just at the start of the age of AI, multimodal AIs, enterprise AI sovereign AI and physical AI are right around the corner. We will grow strongly in 2025.
Going forward, data centers will dedicate most of CapEx to accelerated computing and AI. Data centers will increasingly become AI factories and every company will have either renting or self-operated.
I want to thank all of you for joining us today. I'm joining us at GTC in a couple of weeks. We're going to be talking about Blackwell Ultra, Rubin and other new computing, networking, reasoning AI, physical AI products. and a whole bunch more. Thank you.
This concludes today's conference call. You may now disconnect.